Authors: Michaela Lebedikova, Michal Tkaczyk, Jana Blahosova, Steriani Elavsky, David Smahel
Please cite as: Lebedikova, M. et al. (2022): Researching adolescents’ digital technology usage with a smartphone-based ecological momentary assessment (EMA). In S. Kotilainen (Ed.), Methods in practice: Studying children and youth online (chapter 2). Retrieved DD Month YYYY, from https://core-evidence.eu/methods-toolkit/handbook-part1/handbook-digital-technology-ema, doi: https://doi.org/10.21241/ssoar.83031.

Video: Talking abstract - Researching adolescents’ digital technology usage with a smartphone-based ecological momentary assessment (EMA)
If you are experiencing issues with the video player, please watch the video here on our YouTube channel. We are in the process of fixing this issue. Please excuse the inconvenience.
Children and youth spend increasingly more time on their smartphones, which significantly impacts their lives (George & Odgers, 2015). The current understanding of adolescents’ smartphone usage is limited, relying primarily on self-report surveys, and qualitative research (Ólafsson, Livingstone & Haddon, 2014). Present research would benefit from new methods with high ecological validity that allow capturing the details about digital technology usage over time.
Ecological momentary assessment (EMA) methods may provide answers. EMA is aimed at studying daily lives and detailed temporal variability in experiences, moods, and behaviours in the natural environment. EMA involves intensive repeated data sampling (i.e., several times a day), allowing for the examination of both within- and between-person variability with both reduced retrospective recall and self-report bias (Heron et al., 2017). Recent developments and the ubiquity of smartphones among adolescents have allowed for sampling from adolescents‘ own phones (as opposed to researcher-supplied phones) to study authentic smartphone usage (Domoff et al., 2021, Ram et al., 2019). The self-reported data can be combined with objective data from smartphones or wearable devices to create a detailed and informationally rich picture of adolescents’ smartphone usage and its impacts.
In our project, we aimed to fill this gap and developed an app to investigate the impact of smartphone usage on adolescents’ well-being (IRTIS, 2021). Our study involves four measurement bursts spaced over one year. Each burst is 14 days long, with self-reported sampling four times a day. We combine the self-reported data with objective smartphone data logs and screenshot collections. To ensure the privacy of our participants, we developed machine-learning-based anonymization software (see the detail for how it works in Figure 1). The app development took two and half years and involved several pilot studies and in-house tests. The app is available only for Android devices and some technical problems persist on certain types of devices (e.g., the problems are mostly related to questionnaire notifications and battery optimization on Huawei and Xiaomi devices). Despite these technical issues, our first measurement burst with 201 participants (aged 13 to 17) had an overall compliance rate of 73% (for successfully notified questionnaires), which is an acceptable result and in line with other EMA studies with youth, which have average compliance of 76% (Heron et al., 2017).
Our experience suggests that the EMA method can be successfully applied by combining self-reported data with objective data from mobile phones, allowing for a unique understanding of adolescents’ smartphone behaviour and the complex temporality of effects at both the within- and between-person levels. However, there are downsides to this approach. First, it is challenging to adapt the study design to fit school schedules. Second, despite developing anonymization software and having the approval of relevant ethical bodies, some participants reported distrust and intrusion upon their privacy as reasons for non-participation. Third, our app is downloadable through Google Play, where we faced privacy policy limitations to our data collection (e. g., Google Play unexpectedly removed our app several months after initial approval, forcing us to disable GPS logging component).
In terms of future directions, we plan to incorporate machine-learning approaches for the detection of risky behaviour (e.g., exposure to sexually explicit content). Concretely, screenshots will be processed using the optical character recognition (OCR) software we are developing. The data will be then automatically anonymized (Sotolar, Plhak & Smahel, 2021) and a machine learning text classifier will be developed to facilitate real-time automatic classification of specific behaviours. Our preliminary findings, based on anonymized Messenger conversations provided by participants of the pilot studies, show promising results for the classification of online risks and online supportive interactions.
Lessons learned:
Despite some drawbacks, EMA is a suitable and feasible method (see Heron et al., 2017) for overcoming the limitations of the current research on adolescents’ technology use. For researchers who consider using similar study designs, we underscore the importance of having a strong team of software developers for the entire duration of the project, as various technical issues and limitations emerge at all stages of the research process. Second, we advise accounting for difficulties with both participation and adherence (lower participation rate and higher dropout rate than typically reported in studies with researcher-provided smartphones) due to privacy concerns and burdensome study protocol. Third, as this type of research requires significant privacy intrusion and involves third-party data, careful consideration of the legal and ethical ramifications remains a key necessity.
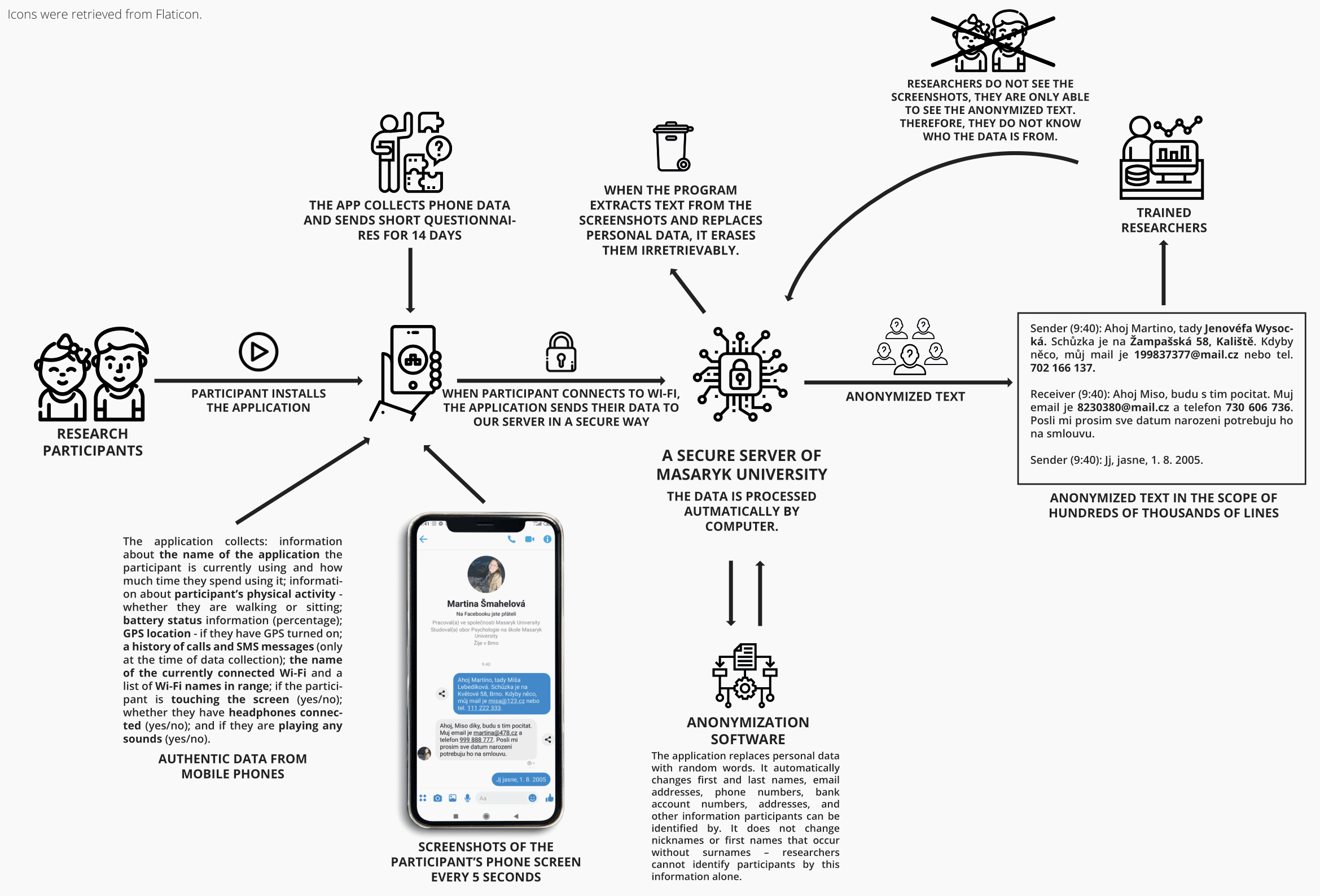
Figure 1. Explanation of our study of adolescent smartphone behaviour.
-
Domoff, S. E., Banga, C. A., Borgen, A. L. Foley, R. P., Robinson, C., Avery, K., & Gentile, D. A. (2021). Use of passive sensing to quantify adolescent mobile device usage: Feasibility, acceptability, and preliminary validation of the eMoodie application. Human Behavior & Emerging Technologies, 3, 63– 74. https://doi.org/10.1002/hbe2.247
-
George, M. J., & Odgers, C. L. (2015). Seven Fears and the Science of How Mobile Technologies May Be Influencing Adolescents in the Digital Age. Perspectives on Psychological Science, 10(6), 832–851. https://doi.org/10.1093/jpepsy/jsx078
-
Heron, K. E., Everhart, R. S., McHale, S. M., & Smyth, J. M. (2017). Using Mobile-Technology-Based Ecological Momentary Assessment (EMA) Methods with Youth: A Systematic Review and Recommendations. Journal of Pediatric Psychology, 42(10), 1087–1107. https://doi.org/10.1093/jpepsy/jsx078
-
IRTIS. (2021). Adolescents on smartphones. Interdisciplinary Research Team on Internet and Society. https://irtis.muni.cz/working-groups/adolescents-on-smartphones
-
Ólafsson, K., Livingstone, S., & Haddon, L. (2014). Children’s Use of Online Technologies in Europe. A review of the European evidence base. LSE, London: EU Kids Online. Revised edition.
-
Ram, N., Yang, X., Cho, M.-J., Brinberg, M., Muirhead, F., Reeves, B., & Robinson, T. N. (2020). Screenomics: A New Approach for Observing and Studying Individuals’ Digital Lives. Journal of Adolescent Research, 35(1), 16–50. https://doi.org/10.1177/0743558419883362
-
Sotolář, O., Plhák, J., Šmahel, D. (2021). Towards Personal Data Anonymization for Social Messaging. In: TSD 2021. LNCS (LNAI), vol. 12848. Springer, Cham.